A New Data-Driven Model to Predict Monthly Runoff at Watershed Scale: Insights from Deep Learning Method Applied in Data-Driven Model
Document Type
Article
Publication Date
10-1-2024
Abstract
Accurate forecasting of mid to long-term runoff is essential for water resources management. However, the traditional model cannot predict well and the precision of runoff forecast needs to be further improved. Here, we proposed a novel data-driven model aimed at enhancing the performance of the Gated Recurrent Unit (GRU) through the integration of Robust Local Mean Decomposition (RLMD) and the Slime Mould Algorithm (SMA). The objective is to improve mid to long-term runoff prediction in three hydrographic stations: Heishiguan, Baimasi, and Longmenzhen, located within the Yiluo River Watershed in central China. The model leverages monthly runoff data spanning from 2007 to 2022 to achieve this objective. The results indicated that (1) the new data-driven model (RLMD -SMA-GRU) had the highest monthly runoff prediction accuracy. Both RLMD and SMA can improve the accuracy of the model (NSE = 0.9466). (2) The precision of the models in wet season outperformed in dry season. (3) The hydrological stations with large discharge and stable runoff sequence have better forecasting effect. The RLMD-SMA-GRU model has good applicability and can be applied to the monthly runoff forecast at watershed scale.
Publication Source (Journal or Book title)
Water Resources Management
First Page
5179
Last Page
5194
Recommended Citation
Jia, S., Wang, X., Xu, Y., Liu, Z., & Mao, B. (2024). A New Data-Driven Model to Predict Monthly Runoff at Watershed Scale: Insights from Deep Learning Method Applied in Data-Driven Model. Water Resources Management, 38 (13), 5179-5194. https://doi.org/10.1007/s11269-024-03907-8
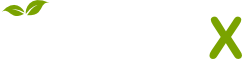
- Citations
- Citation Indexes: 1
- Usage
- Downloads: 6
- Abstract Views: 1
- Captures
- Readers: 9
- Mentions
- News Mentions: 1