Integrated sensing and machine learning: Predicting saccharine and bioenergy feedstocks in sugarcane
Document Type
Article
Publication Date
9-1-2024
Abstract
Predicting saccharine and bioenergy feedstocks in sugarcane enables growers and industries to determine the precise time and location for harvesting a better-quality product in the field. On one hand, Brix, Purity, and total recoverable sugars (TRS) can provide meaningful and reliable indicators of high-quality raw materials for first-generation (1 G) bioethanol. Conversely, Cellulose, Hemicellulose, and Lignin are the primary constituents of straw, directly contributing to second-generation (2G) bioethanol. However, analyzing these materials in the laboratory is a time-consuming and non-scalable task. Therefore, we propose an approach based on a multi-sensor framework, which includes multispectral unmanned aerial vehicle (UAV) imagery, thermal, photosynthetic active radiation (PAR), and chlorophyll fluorescence (ChlF) data, along with machine learning (ML) algorithms namely random forest (RF), multiple linear regression (MLR), decision tree (DT), and support vector machine (SVM), to develop a non-invasive and predictive framework for mapping sugarcane feedstocks. We collected samples of stalks and leaves/straw during the maturity stage while simultaneously collecting remote sensing data. The ML models played a crucial role in predicting 1 G (R2 = 0.88–0.93) and 2 G (R2 = 0.56–0.82) feedstocks. Notably, remote sensing data could serve as important features for the models, mainly through the spectral bands (Blue, Green, and RedEdge), DTemp and ChlF. Hence, the best features can be further implemented within a framework to predict sugarcane feedstocks. Our study marks a significant advancement in the industrial-scale prediction of sugarcane feedstocks, providing stakeholders with invaluable prescriptive harvesting strategies for both primary products and by-products.
Publication Source (Journal or Book title)
Industrial Crops and Products
Recommended Citation
Barbosa Júnior, M., Moreira, B., Duron, D., Setiyono, T., Shiratsuchi, L., & Silva, R. (2024). Integrated sensing and machine learning: Predicting saccharine and bioenergy feedstocks in sugarcane. Industrial Crops and Products, 215 https://doi.org/10.1016/j.indcrop.2024.118627
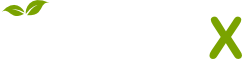
- Citations
- Citation Indexes: 3
- Usage
- Abstract Views: 4
- Captures
- Readers: 21
- Mentions
- News Mentions: 1