Document Type
Article
Publication Date
1-1-2009
Abstract
Predictive models of optimum sweetpotato (Ipomoea batatas) harvest in relation to growing degree days (GDD) will benefit producers and researchers by ensuring maximum yields and high quality. A GDD system has not been previously characterized for sweetpotato grown in Louisiana. We used a data set of 116 planting dates and used a combination of minimum CV, linear regression (LR), and several algorithms in a data mining (DM) mode to identify candidate methods of estimating relationships between GDD and harvest dates. These DM algorithms included neural networks, support vector machine, multivariate adaptive regression splines, regression trees, and generalized linear models. We then used candidate GDD methods along with agrometeorological variables to model US#1 yield using LR and DM methodology. A multivariable LR model with the best adjusted r2 was based on GDD calculated using this method: maximum daily temperature (Tmax) - base temperature (B), where if Tmax > ceiling temperature [C (90°F)], then Tmax = C, and where GDD = 0 if minimum daily temperature <60°F. The following climate-related variables contributed to the improvement of adjusted r2 of the LR model: mean relative humidity 20 days after transplanting (DAT), maximum air temperature 20 DAT, and maximum soil temperature 10 DAT (log 10 transformed). In the DM mode, this GDD method and the LR model also demonstrated high predictive accuracy as quantified using mean square error. Using this model, we propose to schedule test harvests at GDD = 2600. The harvest date can further be optimized by predicting US#1 yield using GDD in combination with climate-based predictor variables measured within 20 DAT.
Publication Source (Journal or Book title)
HortTechnology
First Page
133
Last Page
144
Recommended Citation
Villordon, A., Clark, C., Ferrin, D., & LaBonte, D. (2009). Using growing degree days, agrometeorological variables, linear regression, and data mining methods to help improve prediction of sweetpotato harvest date in louisiana. HortTechnology, 19 (1), 133-144. https://doi.org/10.21273/hortsci.19.1.133
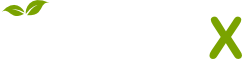
- Citations
- Citation Indexes: 43
- Policy Citations: 1
- Usage
- Downloads: 5
- Abstract Views: 3
- Captures
- Readers: 63