Deep learning based approaches from semantic point clouds to semantic BIM models for heritage digital twin
Document Type
Article
Publication Date
12-1-2024
Abstract
This study focuses on the application of deep learning for transforming semantic point clouds into semantic Building Information Models (BIM) to create a Heritage Digital Twin, centering on Taoping Village, a site of historical and cultural significance in Sichuan, China. Utilizing advanced technologies such as unmanned aerial vehicles and terrestrial laser scanning, we capture detailed point cloud data of the village. A pivotal element of our methodology is the KP-SG neural network, which exhibits outstanding overall performance, particularly excelling in accurately identifying 11 categories. Among those categories, buildings and vegetation, achieves recognition rates of 81% and 83% respectively, and a 2.53% improvement in mIoU compared to KP-FCNN. This accuracy is critical for constructing detailed and accurate semantic BIM models of Taoping Village, facilitating comprehensive architecture and landscape analysis. Additionally, the KP-SG’s superior segmentation capability contributes to the creation of high-fidelity 3D models, enriching virtual reality experiences. We also introduce a digital twin platform that integrates diverse datasets, their semantic information, and visualization tools. This platform is designed to support process automation and decision-making and provide immersive experiences for tourists. Our approach, integrating semantic BIM models and a digital twin platform, marks a significant advancement in preserving and understanding traditional villages like Taoping and demonstrates the transformative potential of deep learning in cultural heritage conservation.
Publication Source (Journal or Book title)
Heritage Science
Recommended Citation
Pan, X., Lin, Q., Ye, S., Li, L., Guo, L., & Harmon, B. (2024). Deep learning based approaches from semantic point clouds to semantic BIM models for heritage digital twin. Heritage Science, 12 (1) https://doi.org/10.1186/s40494-024-01179-4
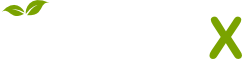
- Citations
- Citation Indexes: 9
- Usage
- Downloads: 11
- Abstract Views: 1
- Captures
- Readers: 89
- Mentions
- News Mentions: 1