Hardware Accelerator for Adversarial Attacks on Deep Learning Neural Networks
Document Type
Conference Proceeding
Publication Date
10-1-2019
Abstract
Recent studies identify that Deep learning Neural Networks (DNNs) are vulnerable to subtle perturbations, which are not perceptible to human visual system but can fool the DNN models and lead to wrong outputs. A class of adversarial attack network algorithms has been proposed to generate robust physical perturbations under different circumstance. These algorithms are the first efforts to move forward secure deep learning by providing an avenue to train future defense networks, however, the intrinsic complexity of them prevents their broader usage.In this paper, we propose the first hardware accelerator for adversarial attacks based on memristor crossbar arrays. Our design significantly improves the throughput of a visual adversarial perturbation system, which can further improve the robustness and security of future deep learning systems. Based on the algorithm uniqueness, we propose four implementations for the adversarial attack accelerator (A3) to improve the throughput, energy efficiency, and computational efficiency.
Publication Source (Journal or Book title)
2019 10th International Green and Sustainable Computing Conference, IGSC 2019
Recommended Citation
Guo, H., Peng, L., Zhang, J., Qi, F., & Duan, L. (2019). Hardware Accelerator for Adversarial Attacks on Deep Learning Neural Networks. 2019 10th International Green and Sustainable Computing Conference, IGSC 2019 https://doi.org/10.1109/IGSC48788.2019.8957192
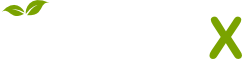
- Citations
- Citation Indexes: 6
- Patent Family Citations: 1
- Usage
- Abstract Views: 1
- Captures
- Readers: 15