Artificial intelligence to guide precision anticancer therapy with multitargeted kinase inhibitors
Document Type
Article
Publication Date
11-24-2022
Abstract
BACKGROUND: Vast amounts of rapidly accumulating biological data related to cancer and a remarkable progress in the field of artificial intelligence (AI) have paved the way for precision oncology. Our recent contribution to this area of research is CancerOmicsNet, an AI-based system to predict the therapeutic effects of multitargeted kinase inhibitors across various cancers. This approach was previously demonstrated to outperform other deep learning methods, graph kernel models, molecular docking, and drug binding pocket matching. METHODS: CancerOmicsNet integrates multiple heterogeneous data by utilizing a deep graph learning model with sophisticated attention propagation mechanisms to extract highly predictive features from cancer-specific networks. The AI-based system was devised to provide more accurate and robust predictions than data-driven therapeutic discovery using gene signature reversion. RESULTS: Selected CancerOmicsNet predictions obtained for "unseen" data are positively validated against the biomedical literature and by live-cell time course inhibition assays performed against breast, pancreatic, and prostate cancer cell lines. Encouragingly, six molecules exhibited dose-dependent antiproliferative activities, with pan-CDK inhibitor JNJ-7706621 and Src inhibitor PP1 being the most potent against the pancreatic cancer cell line Panc 04.03. CONCLUSIONS: CancerOmicsNet is a promising AI-based platform to help guide the development of new approaches in precision oncology involving a variety of tumor types and therapeutics.
Publication Source (Journal or Book title)
BMC cancer
First Page
1211
Recommended Citation
Singha, M., Pu, L., Stanfield, B. A., Uche, I. K., Rider, P. J., Kousoulas, K. G., Ramanujam, J., & Brylinski, M. (2022). Artificial intelligence to guide precision anticancer therapy with multitargeted kinase inhibitors. BMC cancer, 22 (1), 1211. https://doi.org/10.1186/s12885-022-10293-0
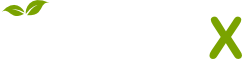
- Citations
- Citation Indexes: 6
- Usage
- Abstract Views: 10
- Captures
- Readers: 32
- Mentions
- News Mentions: 2